We all know the dilemma. We take our suitcase and pack for our upcoming trip. The suitcase looks huge when we start packing. But in no time, it is crammed. There are still a few things that we’d like to carry, but there is no more space. We need to do two things now: pack the existing things compactly so that they take up less space, thus creating space for new additions. Or remove things which are unnecessary, so that we have space for what we find necessary.
We face a similar dilemma in computing. We encounter low disk space or are stuck with low bandwidth and have huge files to store or transfer. How do we solve the problem? The answer is data compression. We will learn about two compression methodologies: lossy and lossless data compression.
The principle behind data compression
We all like to fit more in less. That’s the idea behind data compression. It solves the challenge of fitting more data into less number of bytes so that we get more bang for the buck in terms of storage and bandwidth.
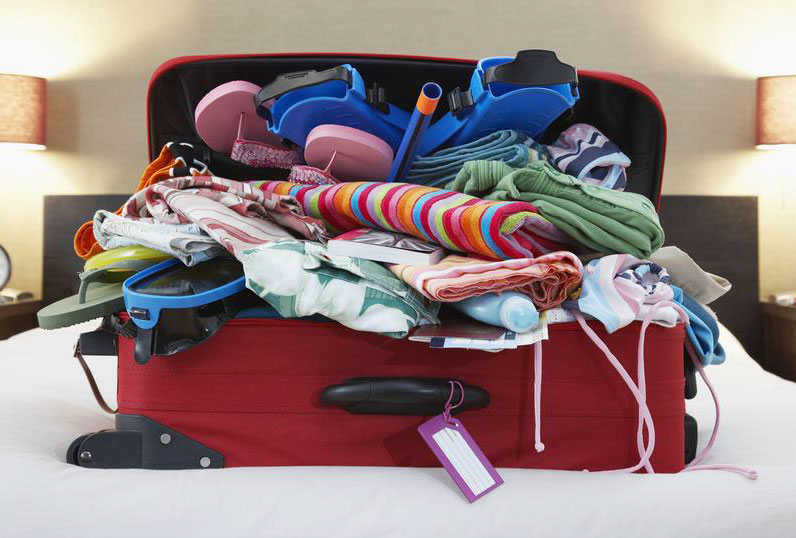
The key principles behind data compression are
- Remove the unnecessary
- Compact what remains as much as possible.
Based on whether an algorithm removes data before compression, there are two approaches: Lossy and Lossless.
Lossless method compresses data without eliminating any of the original data in the process. This can be likened to folding clothes more compactly in a suitcase so that each item occupies less space, thus freeing up space for more.
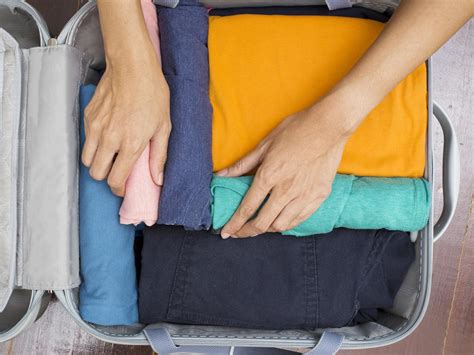
Lossy method deliberately discards data that is not useful. It also eliminates data that can be inferred or reconstructed. E.g. if we were to discard our shaving kit from our suitcase, we can always avail of a barber’s service in a well-equipped city.
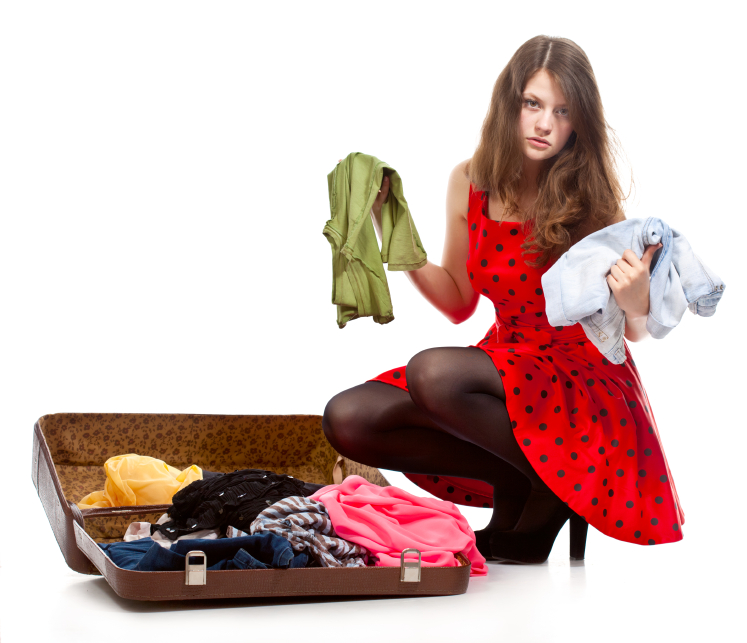
Let us look at how each compression method works in practice and analogies.
Lossless compression by evaluating the required space
This method evaluates if a piece of data can take less space than the default method. What does that mean?
When we buy a notebook, we pick one of the standard sizes, i.e. letter, A4, A5, etc. There are 100 or 200 pages in a notebook. Each of the pages is exactly the same size. E.g. every page on my notebook has 23 ruled lines. But how much do I actually write on?
Out of 23 ruled lines, I probably use about 13-14 on average. I leave blank lines as space between different points. If my content fits before I can use 23 lines, then the rest of the page is left unused. New topic, new page. Look at the image below. I have used just 3 and a half lines out of 23 on a page in my notebook. The remaining 20 lines are useless for this content.
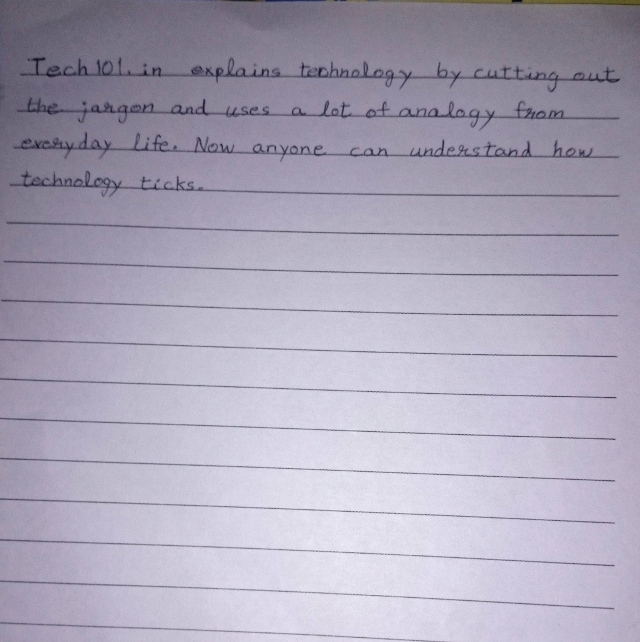
How can I fit this content into something that will fit my pocket? It is possible, but I need to work on it. E.g. I can use a pocket notebook and start writing my content end-to-end with minimal line spacing and by not giving page breaks between different topics. Alternatively, I can start cut out paper bits from my original notebook and start clipping them on a large foldable sheet of paper or a scrapbook. I can keep the sheet folded in my pocket. Either way, I have compressed the content of my notebook into a form-factor that fits my pocket.
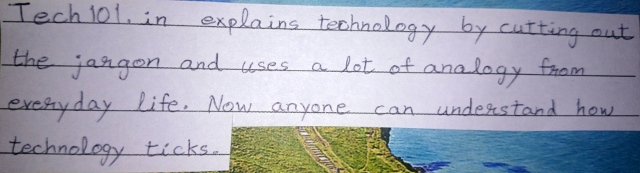
The notebook manufacturer makes a one-size-fits-all solution, but you can plan a way to compress your content into a size you desire.
Computers use data compression in a similar fashion. Each letter of the alphabet occupies a whopping 2 bytes (or 16 bits) of storage! Why? Well, a computer has no way of knowing beforehand which language you’ll use or which characters from those languages you’ll use. To accommodate the possibility of receiving any character from any language, a computer be ready to accommodate more than 60000 different characters. Thus each character requires to be 16 bits or 2 bytes long. This is like the notebook manufacturer who makes a generic A4 sized notebook of 100 pages.
An English document uses small and capital alphabets, numerals and special characters such as brackets, hyphens, spaces, etc. The number of possible characters is about 70 (52 alphabets, 10 numerals and a few special characters). This means that each character can be represented in only 7 bits, which is less than 1 byte! When a lossless data compression algorithm goes through the document, it realises that only 7 bits are required to represent every possible character within the document. It hacks away the unnecessary bits from every character in the document. What used to occupy 16 storage units, now uses only 7. The data is reduced to 7/16th or 44% of its original size.
Lossless compression by clubbing repeated data
Consider the phone number, 9813322257.
Here is one way to say it: Nine, Eight, One, Three, Three, Two, Two, Two, Five, Seven.
And here’s another: Nine, Eight, One, Double Three, Triple Two, Five, Seven.
While speaking, you will find that the second way takes far less time.
Another example: If 5 persons eat at a restaurant, but three of them order coffee, we never say, “coffee for him, coffee for her, coffee for her”. It would always be, “Three coffees”.
Clubbing repeated information to say, “n times this” is a very powerful way to compress things. Data compression algorithms are excellent at clubbing repeated data. Text documents rarely see repeating patterns, but sound and image data see them in big chunks. They hugely benefit from this type of compression. We will look at detailed examples later.
Lossy compression
Lossy compression relies on the fact that data has irrelevant chunks that can be discarded so that what remains is still completely usable and makes sense. Lossy compression must be extremely smart about what to discard. It is hard to program a perfect lossy algorithm.
Instead of pure maths, lossy algorithms take advantage of other factors like human biology and context, to get rid of irrelevant data.
Here is a very simple example.
Question: “What is the colour of the shirt you are wearing?”
Answer 1: “The colour of the shirt I am wearing is red.”
Answer 2: “Red”
Answer 2 is one-worded, but it completely conveys the meaning that answer 1 does. The continuity of the conversation provides context. So the answer eliminates everything other than the information needed. Just one word.
But it does not always work.
Question over phone: “What are you wearing to the party tonight?”
Answer 1: “My red evening gown.”
Answer 2: “Red”
It makes no sense to compress the answer to one word since the term red does not fully convey the complete message.
A lossy compression algorithm evaluates if certain pieces of data can be discarded, but also ensures that what remains is still as useful as the original data itself.
Data compression examples
Two of the best applications, where both lossy and lossless compression are used together, are JPEG image format and MP3 audio format.
JPEG image
Lossy part: In JPEG images, continuous blocks having similar shades of colour are converted to an average same shade. These changes are undecipherable to the human eye. E.g. In photos, a camera lens often captures different shades of blue in a clear sky, but the human eye is unable to spot most of the differences, except for the ones near the horizon. JPEG uses this human limitation to flatten multiple shades of blue into a single average blue.
Lossless part: After flattening similar shades to a single one, it is possible to compress the data by reducing repetition.
E.g. If the sky takes up a section of the photo that is 1000 x 50 pixels starting from the top of the image, the original data would have mentioned a table of rows and column, each cell representing a pixel inside the photo. Each cell contains the shade of blue to be painted in that pixel. But compressed data simply says, “Draw a block of 1000 x 50 pixels of a particular single shade of blue starting from the top left of the image.”
How does that achieve compression? A coloured pixel can be represented by 3 bytes, one byte for red, one for green and one for blue. These three primary colours represent every colour that can be seen on a screen. In an uncompressed image, an area of 1000 x 50 pixels is thus represented by 1000 x 50 x 3, which is 150,000 bytes.
In a compressed image which reduces repetition, 3 bytes are used to store the colour, 4 bytes to mention from which pixel in the image to start painting that colour, and 4 bytes to mention where to stop, thus taking only 3 + 4 + 4 = 11 bytes to represent the entire blue block of the sky.
11 bytes against 150,000. This is a reduction by a factor of more than 10,000!
MP3 audio
Lossy part: In MP3, all the sounds captured by the mic, but not audible to the human ears are first removed. A human ear is only capable of hearing sounds within the frequencies of 20 Hz to 20 kHz. But a mic can record frequencies not audible to the human ear. An outdated sound format such as WAV stores those frequencies too.
Next, all the sound notes that are too close in frequencies for the human ear to distinguish are averaged into the same frequency.
Lossless part: Repeating patterns are identified. E.g. a guitar riff that repeats throughout the song. One can extract the patterns and specify how many times to repeat them in the track. E.g. This drum rhythm is to be played repeatedly from the 38th second to the 140th second of the track. Or this keyboard sequence is to be played from the 41st second and repeated 30 times.
After performing the lossy and lossless phases, an incredible amount of compression is achieved. Despite several chunks of data thrown away as part of lossy compression, the image or the sound makes perfect sense to the user, because of the limitations in eyesight and hearing.
Conclusion
With the increasing ability of computers to handle different types of content and the bigger and heavier they get, it is absolutely vital to have a solid data compression plan in place, so that content can be stored in as little space as possible and can be transferred across networks, incurring as little cost and delay as possible.
Further reading
- Blog post: How does data compression work? on NetMotion
- Book: Data compression by David Salomon (Buy on Amazon.in | Amazon.com)
Wow..that’s a super simple and super cool explanations. I understand now why for some files .zip make a huge difference and for some it makes no difference at all. Way to go !!!
Thank Priya. Yes indeed, the data itself makes a lot of difference on how well it is compressed. It is all mathematics 🙂